本資料は2020年7月9日に社内共有資料として展開していたものをWEBページ向けにリニューアルした内容になります。
■Outline
- Introduction
- Definition of the problem
- Challenges
- Common approaches
- Statistical body models
- Definition of the setup
- Aim of the methods
- Typical solutions
- SMPL
- 3D human pose
- Challenges
- 2D solutions
- SMPLify
- Conclusions
■The data
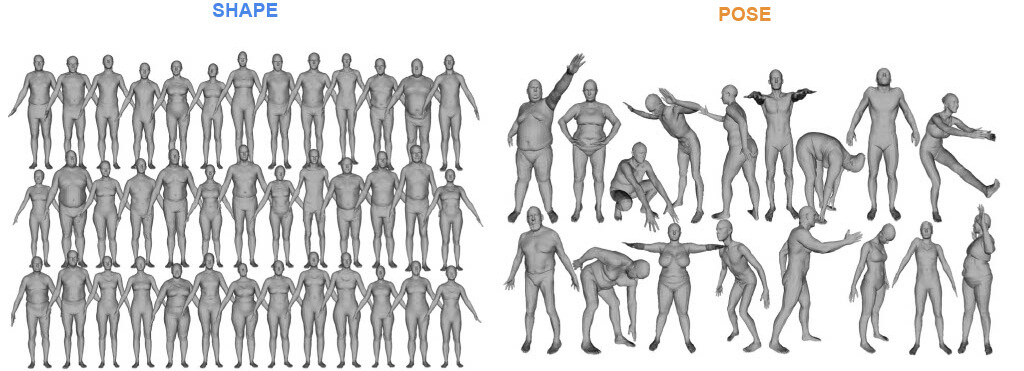
■The Model

■SMPL
SMPL stands for Skinned Multi-Person Linear model.
It is a “statistical” body model, which means it is learned from data.
It captures 2 aspects of the human body:
- Shape
- Pose

■Example: Joint regression

■Example: Inference
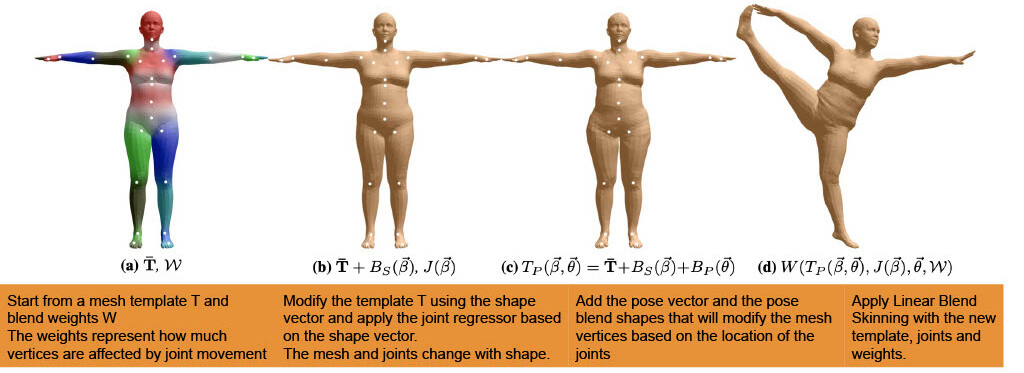
■SMPLify
The goal of SMPLify is to automatically estimate
both 3D pose and 3D body shape from a single RGB image.

■Ingredients
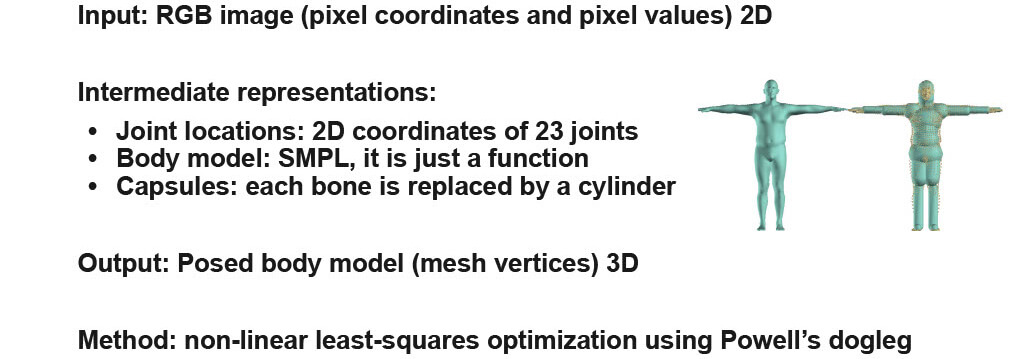
■Objective functions
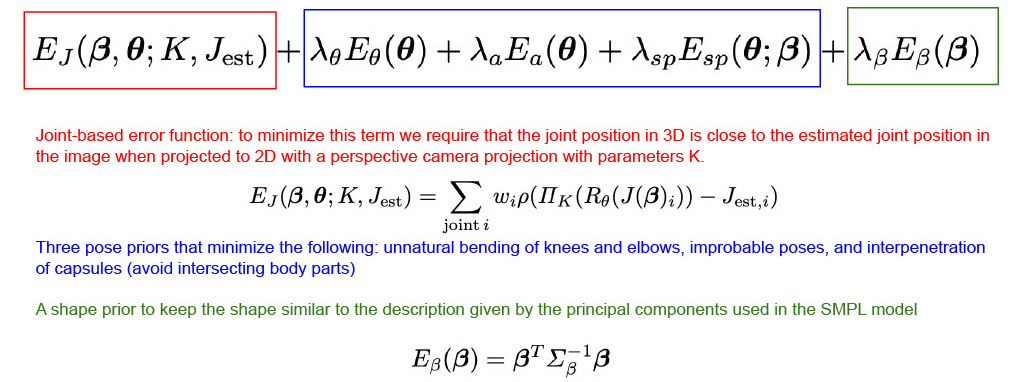
■Example: comparison

■References
Models
- SMPL
- Project website: https://smpl.is.tue.mpg.de/
- SMPLify
- Project website: http://smplify.is.tue.mpg.de/
Datasets
- FAUST
- Project website: http://faust.is.tue.mpg.de/
- CAESAR
- Project website: http://store.sae.org/caesar/
My ongoing notes on Notion:
https://www.notion.so/erinaldi/Fundamentals-of-riggin g-eb8acd313a444b6999ef1b0a7a13b892
■Future discussion
A few links for modern motion capture papers:
- DeepCap:
Monocular Human Performance Capture Using Weak Supervision, CVPR 2020 - EventCap:
Monocular 3D Capture of High-Speed Human Motions using an Event Camera, CVPR 2020 - HandCap:
Monocular Real-time Hand Shape and Motion Capture using Multi-modal Data, CVPR 2020 - StyleRig:
http://gvv.mpi-inf.mpg.de/projects/StyleRig/ - XNect:
Real-time Multi-Person 3D Motion Capture with a Single RGB Camera, SIGGRAPH 2020 - LiveCap:
Real-time Human Performance Capture from Monocular Video, ToG 2019 - MonoPerfCap:
Human Performance Capture from Monocular Video, TOG 2018